WiDS REGENSBURG 2022
July 5th and 6th 2022 at Degginger, Regensburg (hybrid event)
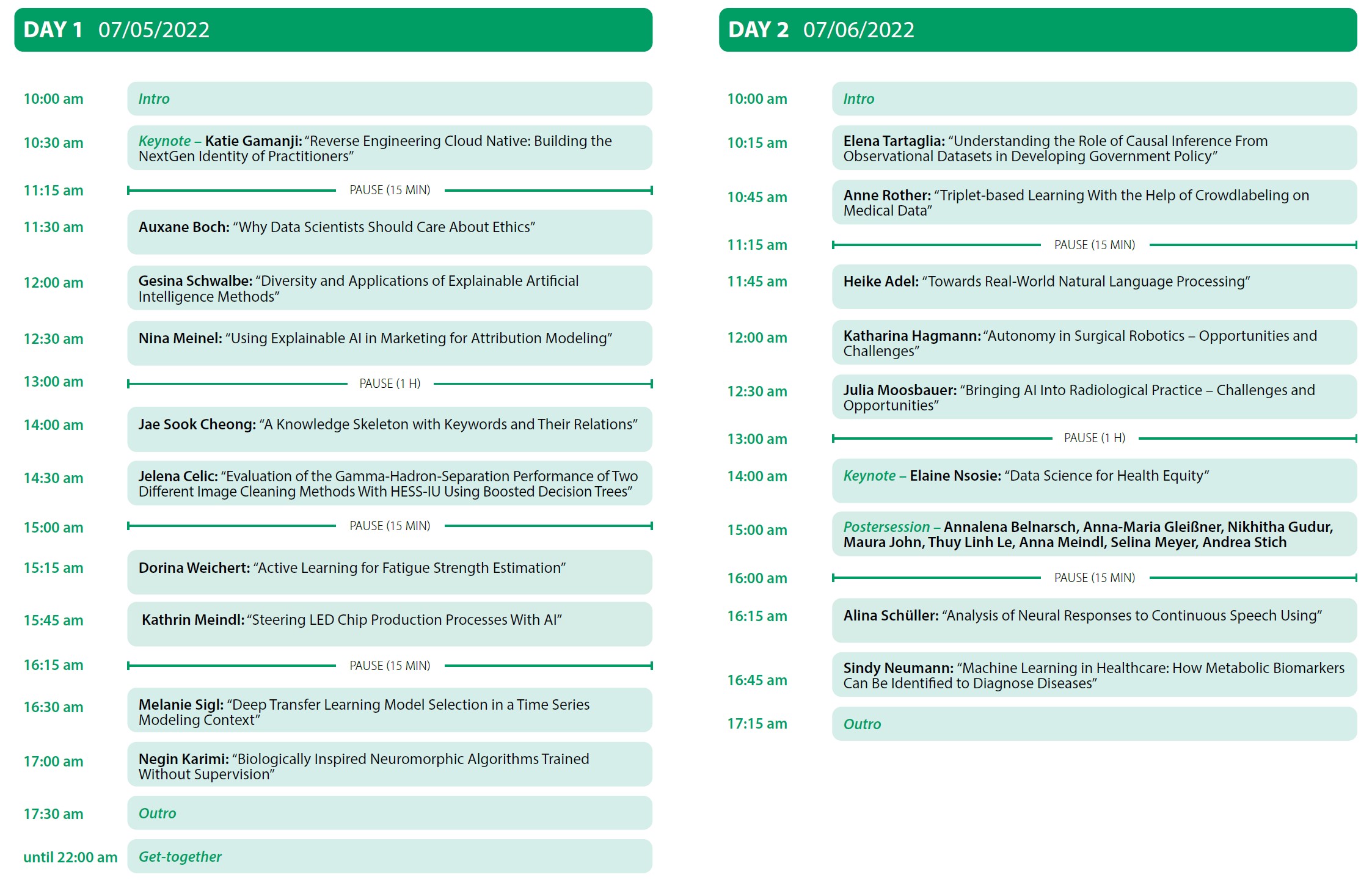
MATERIAL (2022)
Recordings:
https://youtu.be/2674w93U65k (Hagmann)
https://youtu.be/34cSVa-CbOA (Adel)
https://youtu.be/Gfm0OGuZjFY (Tartaglia)
https://youtu.be/ewpDt-UNYNE (Karimi)
https://youtu.be/ZOTqJkXzpE0 (Meindl)
https://youtu.be/_X9wLvyxf20 (Celic)
https://youtu.be/Mr1kzbw2Zaw (Cheong)
https://youtu.be/QJuEsA0zhqo (Schwalbe)
AGENDA
We are proud to have presented an impressive range of talks! The keynotes and technical talks were given by experienced data scientists from both academia and industry. The program was completed by a poster session featuring promising students who want to share their latest data science projects with the audience.
Day 1 (July 5th 2022)
10:00 am: Opening Remarks
10:30 am: Keynote: Reverse Engineering Cloud Native: Building the NextGen Identity of Practitioners by Katie Gamanji (Chief of Future Founders Officer at OpenUK)
Kubernetes has become the default container orchestrator framework, setting the standards for application deployment in a distributed environment. In the past years, numerous tools have been developed to extend Kubernetes capabilities and enhance its features. Simultaneously, the expansion of the technology landscape prompted the growth of the adopter base and the number of scenarios where cloud native can be applied. The organic adoption and development of new tools, created the ecosystem and community as we know it today. This keynote will feature the 3 core principles that define the next generation’s identity of cloud native practitioners using a reverse engineering approach. It will present the interoperability of tools, inclusivity at the community and adopters level, and a culture of change and education that drives the ubiquity of the cloud native.
11:15 am: Break
11:30 am: Why data scientists should care about ethics by Auxane Boch (Technical University of Munich)
Ethics has been defined as reaching for the „good life“ by Socrates. Evolving through time and cultures, today’s ethics applied to technology are composed of a number of principles aiming at making societies better, and protecting populations. In this presentation, we will understand what is ethics in the context of data, why it matters, and especially, why you as a data scientist should care.
12:00 pm: Diversity and Applications of Explainable Artificial Intelligence Methods by Gesina Schwalbe (Continental)
The topic of explainable AI (XAI) methods is getting more into the focus of many application fields. This talk aims to convey the breadth of the research field of XAI, answering: What does/can explainability mean? Where and why is explainability needed? And what kinds of methods are out there to achieve explainability?
12:30 pm: Using Explainable AI in Marketing for Attribution Modeling by Dr. Nina Meinel (Springer Nature)
Attribution Modeling is the holy grail in Marketing and simply, it is the analytical science of determining which marketing tactics are contributing to sales or conversions and understand the user journey. A very common approach is last click, which is easy and understandable. Given the data there is much more a data scientist can do using Machine Learning, Simulation approaches, adding time components and so on. Still the most important is being able to easily interpret the impact of different touchpoints within the journey to truly enable marketers. In the talk we will show how this can be done using a real example.
1:00 pm: Break
2:00 pm: An Knowledge Skeleton with Keywords and their Relations by Dr. Jae Sook Cheong (University of Bayreuth)
Especially in the times when artificial intelligence reshapes human activities and lives, we cannot overstress the importance of learning for humans. Today’s learning is for building a structure of concepts to connect new information, rather than remembering individual information. We propose “Knowledge Structure Graph” representing nodes with concepts with bidirectional weighted edges, to represent evolving knowledge structure. We believe that knowledge structure graphs provide a great framework for effective learning, especially abstract and complicated knowledge, and for discovering new insights.
2:30 pm: Evaluation of the Gamma-Hadron-Separation performance of two different image cleaning methods with HESS-IU using Boosted Decision Trees by Jelena Celic (Erlangen Centre for Astroparticle Physics)
One of the leading experiments in the current generation of Imaging Atmospheric Cherenkov Telescopes (IACTs) and the only IACT on the southern hemisphere is the High Energy Stereoscopic System (H.E.S.S.), an observatory with five telescopes located in Namibia. Even though these experiments are designed to detect gamma-rays, their measurements are dominated by an enormous number of cosmic-ray background events. Therefore, an essential stage in the data analysis is distinguishing between hadron-triggered events and gamma-ray induced events, which can be accomplished using machine learning algorithms. Multivariate analyses combine several event image variables into a single variable that indicates the degree to which an event is identified as gamma-ray-like or cosmic-ray-like. The presentation will describe how boosted decision trees are used as an efficient method for background suppression in gamma-ray astronomy, as well as compare its performance for different image cleaning methods. Besides the standard image cleaning used in the last twenty years, a new time-based image cleaning technique is applied to the event images since the upgrade of the H.E.S.S. Phase one telescopes cameras (HESS-IU). With this method, we retain more information on the event image than with the commonly used one. As a result, better differentiation between background event and signal can be achieved in this data processing step.
3:00 pm: Break
3:15 pm: Active Learning for Fatigue Strength Estimation by Dorina Weichert (Fraunhofer IAIS)
An important material property for metals is fatigue strength. It describes the highest load a probe of this material withstands for a defined number of cycles that is thought to represent an infinite lifetime. Factors like the type of material, its treatment and the type and amount of stress that is applied impact fatigue strength. Additionally, measurements of fatigue strength are noisy: probes with the same features under the same conditions react differently. Experimentally, the estimation of fatigue strength is very costly in terms of monetary expanses and time. Therefore, it is attractive to create a precise estimate with as little experiments as possible. The current state-of-the-art approaches concentrate on the estimation of fatigue strength for a given set of experiments. Only for one of the approaches, the experimental procedure is defined as well, but this still requires hyperparameters. Hence a high level of immediate expert knowledge is required for efficient experimentation in the lab. We propose a new approach, consisting of an experimental procedure and an estimation method for fatigue strength, that reduces the amount of necessary expert knowledge by abstracting it as follows: Given experimental data, we model the general behaviour of the material by a Gaussian Process with a tailored covariance function. We use its prediction as a prior for a maximum a posteriori (MAP) estimate of fatigue strength, where the likelihood reflects the experimental setting. Based on the MAP estimate, we find the experiment with the highest impact on the current estimate and recommend it for the following experiment. A comparison to real life experiments shows that our approach requires less experiments to produce an estimate at the same precision – massively reducing experimental costs.
3:45 pm: Steering LED chip production processes with AI by Kathrin Meindl (ams OSRAM)
The production of a single LED chip involves hundreds of process steps, and small process deviations lead to large deviations in the quality of the output product, e.g. in color or brightness. In general, customer requirements are narrower than the produced distribution of product qualities, which leads to unwanted inventory and scrap. Hence, in order to produce sustainably and economically, it is necessary to decide early in the process which semi-finished products are best suited for which customer order. This presentation will give an overview of the machine learning approach at ams OSRAM to better predict LED chip quality and thus improve the steering of the production.
4:15 pm: Break
4:30 pm: Deep Transfer Learning Model Selection in a Time Series Modeling Context by Melanie Sigl (FAU Erlangen Nuremberg / PRODATO)
Transfer learning is the process of reusing a pre-built deep learning model and transfer its acquired knowledge to a new dataset and task. Research suggests that dataset similarity correlates with positive effects on model performance and a reduce in training time. Thus, selection of an appropriate deep learning model based on dataset similarity is crucial for transfer to avoid a drastic decline in performance. Despite continuous efforts to select an appropriate model to transfer in tasks such as image classification, neural language processing, and univariate time series classification, specifying a dataset similarity measure for time series regression tasks still remains an open research problem. Taking on this problem, we define a similarity measure for transfer in multivariate time series that guides model selection to transfer recurrent neural networks. This talk will give an overview of past and current achievements and ways forward.
5:00 pm: Biologically Inspired Neuromorphic Algorithms Trained without Supervision by Negin Karimi (Technical University of Munich)
Spiking neural networks (SNN) show the closest connection to neurological processes in the brain. Nevertheless they are not yet broadly implemented in industry. We will introduce SNNs with all their advantages and disadvantages and especially tackle the main problem of parametrization. We present a novel approach to use evolutionary optimization and liquid state machines.
5:30 pm: Closing Remarks
6-10 pm: Get Together with Food and Music
No program, just good music, delicious food and plenty of networking opportunity.
Day 2 (July 6th 2022)
10:00 am: Opening Remarks
10:15 am: Understanding the role of causal inference from observational datasets in developing government policy by Dr. Elena Tartaglia (CSIRO – Commonwealth Scientific and Industrial Research Organisation)
One of the aims of public policy is to encourage behaviours towards a desired outcome. To develop effective and evidence-based policy, policymakers need to understand the likely impact of a policy. In other words, they need to understand the causal effect of an intervention. The ‘gold standard’ for showing causal effects is the randomised control trial (RCT). However, there are many situations where RCTs are impossible or unethical. Instead, governments rely on administrative data, which is a type of observational data that typically contains various biases. Controlling for biases is critical when estimating causal effects. Although it is impossible to guarantee perfect bias removal, using causal diagrams and adjustment techniques can help us identify data limitations and better estimate causal effects. I recently worked with the Department of Education, Skills and Employment (DESE) to incorporate causal inference techniques into the analytics underpinning their policy advice and formulation. Example questions of interest to DESE are ‘what is the effect of childcare attendance on student readiness to enter primary school?’ and ‘what is the effect of high school completion on income later in life?’. In this talk, I will highlight the utility of causal inference in policy formulation and address some implementation challenges.
10:45 am: Triplet-based Learning with the Help of Crowdlabeling on Medical Data by Anne Rother (Otto von Guericke University Magdeburg)
As healthcare-related data proliferate, there is need to annotate them expertly for the purposes of personalized medicine. Crowdworking is an alternative to expensive expert labour. Annotation corresponds to diagnosis, so comparing unlabeled records to labeled ones seems more appropriate for crowdworkers without medical expertise. This talk focus on an annotation experiment on health data from a population-based study with hepatic steatosis as example disorder.
11:15 am: Break
11:30 am: Towards Real-World Natural Language Processing by Dr. Heike Adel (Bosch Center for Artificial Intelligence)
State-of-the-art natural language processing models achieve impressive results on well-defined benchmark datasets, yet they are black-box models and often tailored towards a specific domain and language. As such, their generalizability and explainability are still limited. Those aspects, however, are key challenges when models should be deployed in real-world applications where humans play a central role. In this talk, I will first give a brief general introduction of natural language processing and pre-trained language models. Then, I will shed light on two concrete challenges of NLP today – low-resource NLP and explainable NLP – and present our contributions towards real-world natural language processing. In low-resource NLP, the transferability of models to low-resource domains and languages is particularly challenging. For a more effective transfer learning, we have introduced a novel similarity score and method for selecting data sources. In order to be able to make best use of existing resources, we have further proposed a meta-embedding approach for an effective combination of different embeddings. In the context of explainable NLP, it is important to ensure that the provided explanations are actually coupled with the model predictions. To achieve this, we have introduced a novel architecture and regularization term for training the model. Moreover, we have conducted a study that demonstrates that human interpretation of model explanations might be biased and that it is, therefore, important how explanations are presented to users. All those works are examples for steps towards real-world natural language processing. Our experiments and analyses underline the need for careful model training and evaluation.
12:00 pm: Autonomy in surgical robotics – Opportunities and Challenges by Katharina Hagmann (German Aerospace Center)
Minimally Invasive Robotic Surgery (MIRS) has entered modern operating rooms in the last 30 years. Most commercially available robotic systems are telemanipulated by the surgeon. But recent research increases the degree of autonomy of surgical robots from telemanipulation over shard control towards autonomy. Higher levels of autonomy require the aquisition not only of information about the robot but also of environmental information as decision and planning processes are involved. Surgical data science provides environmental information by enabeling advanced imaging technologies like organ segmentation and tracking. This talk adresses the opportunities of surgical data science as well as the challenges of different degrees of autonomy and recent developments.
12:30 pm: Bringing AI into radiological practice – challenges and opportunities by Julia Moosbauer (deepc)
While radiologists are barely able to keep up with the workload due to the increase in exams, recent years‘ research gives hope for relief through AI support. While there are numerous certified and high-quality solutions available, knowledge of how to integrate these solutions into workflows and realize their full clinical value is not yet broadly available. This talk is about the challenges and opportunities in integrating AI solutions into radiology routine.
1:00 pm: Break
2:00 pm: Keynote: Data Science for Health Equity by Prof. Dr. Elaine O. Nsoesie (Boston University)
Data can be used to reduce, eliminate, or worsen health inequity. It is therefore important to understand the factors that impact how a particular dataset is collected and the potential policy impacts of using biased data in developing health interventions. This talk will focus on bias in health data and the need to create new structures that ensure that health data is used to redress health inequity.
3:00 pm: Poster Session (see below for list of posters)
4:00 pm: Break
4:15 pm: Analysis of neural responses to continuous speech using MEG by Alina Schüller (FAU Erlangen Nuremberg)
The human brain processes complex information as it unfolds over time. To understand a spoken sentence, the information has to be continuously processed to build higher level representations from the audio signal to the sentence meaning. Studying the neural dynamics of speech processing in the human brain does not only provide an improved comprehension of the brain function itself, but is substantial for the design of neural protheses such as hearing aids. To improve the understanding of how the human brain represents speech in naturalistic environments and to investigate contributions of subcortical and cortical sources in these neural processings, neuroimaging techniques such as magnetoencephalography (MEG) and electroencephalography (EEG) can be employed. Thanks to its high temporal resolution, both provide insights into the neural processing of continuous stimuli such as speech. The M/EEG response to certain features of speech, e.g. the fundamental frequency corresponding to the pitch of a speaker, can be represented using linear predictors, which are referred to as the Temporal Response Functions (TRFs). One approach to investigate the neural dynamics underlying sensory processing is to study the functional roles of specific components of the TRF, to gather information about behavioral attributes such as attention. In contrast, the cortical origins of the underlying neural processes are not as well understood and methods for source reconstruction address this issue. Both approaches are presented in the talk by means of a MEG dataset, providing neural responses to continuous speech stimuli. Using this dataset, responses to two separate features of the speech stimuli were analyzed: the fundamental frequency, as the carrier of the speech, as well as the corresponding temporal modulations in the spectral envelope of the speech stimulus. TRF analysis yielded a cortical originated peak latency (peak latency provides information about the origin and the auditory pathway of the signal in the brain) at approximately 35 ms, which is in line with the relative insensitivity of MEG recordings to many subcortical structures.
4:45 pm: Machine Learning in Healthcare: how metabolic biomarkers can be identified to diagnose diseases by Dr. Sindy Neumann (Numares)
Metabolomics is the study of small molecules, commonly known as metabolites, within an organism. Nuclear magnetic resonance (NMR) spectroscopy produces a large amount of metabolic data and allows to simultaneously analyze numerous metabolites in a single biological sample such as human urine or serum with one measurement. Because metabolites represent the downstream products of multiple interactions between genes, transcripts, and proteins, they can closely reflect the phenotype of an organism at a given moment. The analysis of metabolic differences between groups of patients enables to gain insights into the underlying disease pathology. To this end, metabolomics has become a valuable instrument in clinical research and the diagnosis of human diseases. Furthermore, machine learning algorithms can be used to explore the complex relationship between metabolic expression and disease followed by the development of diagnostic models that can be applied in the clinical setting. This talk addresses the challenges and opportunities of clinical metabolomics for biomarker discovery in diseases and gives an overview of how metabolic data can be analyzed. In addition, a short insight is given into the regulatory framework for medical devices which governs market access.
5:15 pm: Closing Remarks
Deep Learning in anomaly detection for manufacturing processes by Anna-Maria Gleißner (OTH Regensburg / evopro)
The central task of this thesis is to compare two efficient and robust real-time Deep Learning methods for anomaly detection on component surfaces. Both programs will be able to classify the images containing the objects into „i.O.“ (object ok) and „n.i.O.“ (anomaly detected). On the one hand, YOLOv3 is presented as an example of supervised learning and on the other hand, the fast-AnoGAN method is analyzed, which belongs to semi-supervised learning.
Automated inspection of solder joints in quality control – A deep learning approach by Anna Meindl (OTH Regensburg / ams OSRAM)
This paper utilizes domain knowledge of the soldering process to inspect solder joints in an automated manner by applying Fully Convolutional Networks (FCNs). During the reflow soldering of surface-mounted devices onto printed-circuit boards (PCBs) gas-filled cavities, socalled voids, can occur. These porosities are typically caused by outgassing of flux in the liquid solder and are not visible from the outside without imaging methods like X-ray. Depending on their quantity, size and location, voids can significantly undermine the reliability of solder joints. FCNs are used to determine the voiding level in an automated process and enable further research in this area. The deep learning technique performs a pixel-by-pixel classification of X-ray images of solder joints into the classes solder, voids and background. To obtain well-trained FCNs, a large amount of accurately labeled training data is required. The acquisition of such data sets is often very time-consuming, as it involves a high level of manual effort. Furthermore, it can be difficult to provide the data in sufficient numbers, depending on the application. This work aims to conveniently enhance the amount of training data in an augmentation process based on expert knowledge.
Development of a Federated Learning Based Framework for Lifetime Prediction of Lithium-Ion Batteries by Annalena Belnarsch (TU Munich / AVL SFR)
The lithium-ion battery in any electric vehicle is subject to an inevitable aging process which is difficult to forecast due to complex and chemistry-dependent aging mechanisms. One of the most promising approaches to this problem is the development of data-based aging models using machine learning techniques. A large database with a wide variety of driving conditions has to be collected to train a generalized prediction model that ensures low prediction errors for electric vehicles all over the world. However, collecting such a large database centrally is practically infeasible due to security and data communication restrictions of vehicle fleet operators. Federated learning provides an alternative solution, in which fleet operators can participate in a global training without the need of sharing their private data. In this poster, a concept to incorporate Federated Learning into data-based battery lifetime prediction is presented. In this presented approach, a single artificial neural network is shared across all participating fleet operators. Each operator trains the artificial neural network with its data locally while the weights are adjusted globally based on the learned weights of all fleet operators. Thus, each fleet operator profits from a prediction model that has been trained on a larger and a more versatile database without the need to actually share the raw data with potential competitors.
Reddit data analytics – how data draws attention by Thuy Linh Le (OTH Regensburg / ams OSRAM)
In the world today, data is probably the thing that matters most, exposes inefficiencies as well as opens windows into opportunity. That is why data analysis, which helps to reveal the beauty of the data world we all live in, is gaining so much attention not only from scientists but also from the general public. Analyzing data from the DataIsBeautiful subreddit, where data reaches more than 17 million users, it shows which topics are most relevant and how to illustrate them in the most engaging way for readers. This project is carried out using Natural Language Processing, image analysis methods and finally a Plotly Dashboard containing a model that predicts which post might catch one’s interest and helps to bring the beauty of the data to even more people.
Designing an Empathetic Conversational Agent for Health Behavior Change by Selina Meyer (University of Regensburg)
Changing health behavior is a difficult process that usually requires individuals to change their daily routines and habits. As such it is not only challenging to begin change, but also to maintain it and stay motivated. The poster will outline a potential approach to design a conversational agent to facilitate behavior change by combining psychological frameworks with data science. Specifically, we focus on the potential of natural language processing and generation techniques to assess and increase an individual’s motivation and ability to change. We summarize state-of-the-art language models such as BERT and GPT which were pretrained on huge amounts of text data, outline how they can be adapted to domain-specific data and use cases and explore their potential in the context of this research.
Efficient Permutation-based Genome-wide Association Studies for Normal and Skewed Phenotypic Distributions by Maura John (TU Munich)
Genome-wide Association Studies (GWAS) are the predominant method for studying the complex relationship between genotypes and phenotypic traits. Linear Mixed Model (LMMs) are commonly used to detect associations between genetic markers and the trait of interest, while at the same time allowing to account for population structure and cryptic relatedness. However, assumptions of LMMs include a normal distribution of the residuals and independence of the genetic markers, which are often violated in real data. To overcome some of these limitations, permutation-based methods are useful and provide a more realistic threshold for the detection of true associations. Due to its high computational complexity, they are rarely implemeted in practice. We propose permGWAS, an efficient LMM reformulation based on 3D and 4D-tensors that can provide permutation-based significance thresholds and outperformas current state-of-the-art LMMs with respect to runtime. We show that permutation-based thresholds have lower false-discovery-rate for skewed phenotypes compared to the commonly used Bonferroni threshold.
Architecture study of kernel sizes in Convolutional Neural Networks by Nikhita Gudur (OTH Regensburg)
Multivariate analysis using chemometric data has great significance in various fields. Over the years, various techniques have been found out and applied on chemometrics andamong those, Partial Least Squares (PLS) technique is quite popular and widely being used till date. Recent advancements in the space of Deep Learning (DL) have paved a way for its applications in various fields. It has been observed that Convolutional Neural Networks (CNN) have great scope in the area of chemometrics. However, efficiently training the CNN models by identifying the right set of parameters and hyperparameters has become a major challenge and one of the problems which need to be tackled in order to encourage its application more extensively in chemometrics. In this master thesis work, one such attempt is made at researching and experimenting with various kernel sizes for 1 dimensional CNN models. For different kernel sizes, the variation in error rates has been studied. Models have been trained and evaluated on Mango dataset and the Melamine dataset. Some pre-processing had been required for the datasets before training the models. A set of important hyperparameters have been tuned and kept constant while experimenting with different kernel sizes. During this study, kernel sizes ranging from 2 to 100 has been experimented for both datasets.
Artificial Intelligence@Plattform Lernende Systeme by Andrea Stich (Infineon)
The Learning Systems Platform founded in 2017 by the German Federal Ministry of Education and Research (BMBF) is a network of experts on the topic of Artificial Intelligence (AI). Its goal: to act as an independent broker to promote interdisciplinary exchange and social dialog on AI. The nearly 200 members from science, business and society together with acatech develop positions on opportunities and challenges in working groups and name options for action for the responsible use of AI. As a member of working group 2 „Work/Qualification/Human-Machine Interaction“, Andrea Stich will present examples of the WG 2 results in personal discussion.